Fun, Physics & Machine Learning: A Summer @ CERN Openlab
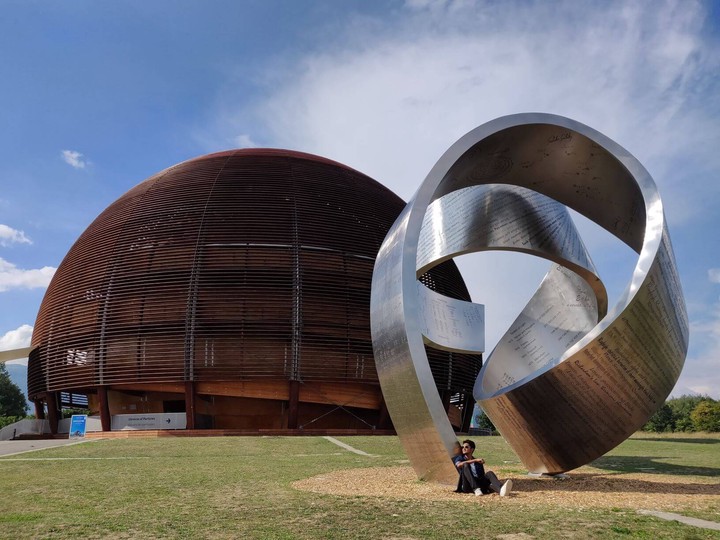
The CERN Openlab ‘18 program is 2 months away. 1 month left. Next week I fly to Geneva. Tomorrow. The first week at CERN. Amazing place. Great people. Challenging work. It’s been a month already. Getting stuck and breaking through. A week left to go. Wrapping up. My last day. Saying goodbyes. Indeed, time flies when you are having fun. My internship at CERN went from being a dream to a memory. From ideas to reality. Problems to possibilities. Foreign strangers to close friends.
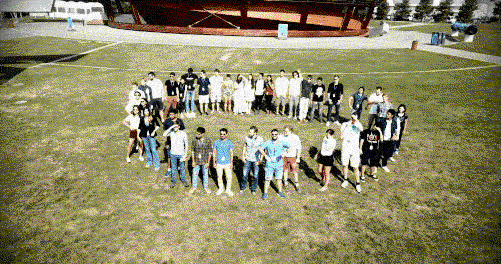
Here I present the condensed highlights of my experiences, in brief of course; for these experiences are enough to fill a lifetime and a rather long-winded blog post.
What immediately strikes one visiting CERN is the unique culture of genuine global collaboration between nations, universities and scientists, driven not by profit margins, but by the noble pursuit of understanding the Universe at the most fundamental level and pushing the boundaries of human knowledge. You stay long enough and you might get to gradually witness the grand endeavour of a diverse international community working towards the shared goal of scientific discovery. It is indeed a humbling experience, one that makes you feel very small and yet significant as a tiny part of something quite so grand.
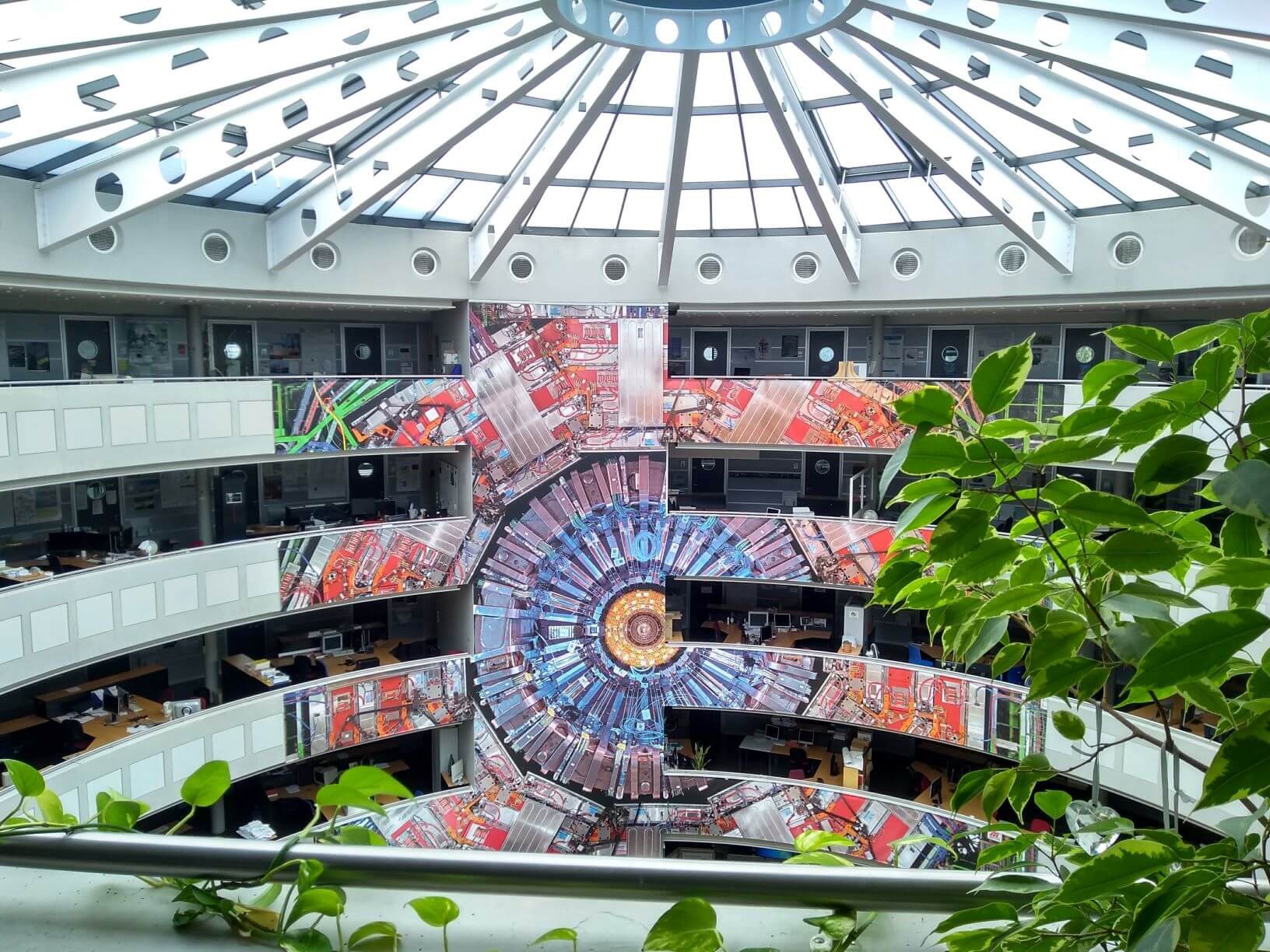
Our entire OpenLab 2018 batch stayed in one apartment hotel. 41 amazing people from 23 different countries under one roof. Yes, the house parties were epic. We had four different nationalities living together in our apartment: Belgian, German, British and Indian. Oh, the Brexit jokes. From silent dance parties to barbeques, from industrial field visits to impromptu Paris trips, afternoon hikes on Swiss mountains to late night deep conversations, we did it all and then some more.
Living together with such a diverse group of people will make one appreciate the cultural differences and yet acutely recognize the universal fabric of humanity that we all share; so different, yet so similar. And sometimes, when I lost sight of it while stuck with a learning model that just would not converge, I just needed to look and ponder at the giant mural of the CMS detector inside the foyer of my office building. Or, take a walk to the “Wandering the Immeasurable” steel sculpture at the Globe of Innovation to know that it is going to be alright:
“…Comprises two stainless steel entwined ribbons that depict a selective chronology of innovations in physics, from the earliest revelations to present experimentation…The sculpture bends in space, ending in mid-air—awaiting the next era of advancement and discovery. Its curve reflects the infinite possibilities presented to us by continual exploration of the complexities that contribute to the fabric of science.”
My work at the CMS Experiment of CERN consisted of researching ways to monitor the trigger system which reduces the event rate of the Large Hadron Collider by discerning possibly interesting events from the usual physical phenomenon. The immense scale of the experimental data made the case for deep learning methods. We tried to use a Variational Autoencoder to learn to reconstruct the trigger rate behaviour but the model failed to converge no matter how many layers we slapped on top of it or tuned the hyperparameters to death. It was evident that now we had to go all in, dive deep and open the black box of deep learning. Having developed the entire learning pipeline using Pytorch, I was able to scrutinize the behaviour of the hidden layers during training. In brief, the prior assumption of a Variational Autoencoder on the latent space distribution was standard Gaussian. Hence, we were failing to take advantage of the cascaded design of the two-level trigger system. Instead, we could use regularization to add a probability constraint on the latent space to learn the Level 1 trigger distribution, modelled as the hidden archetype, given the observable High Level trigger behaviour.
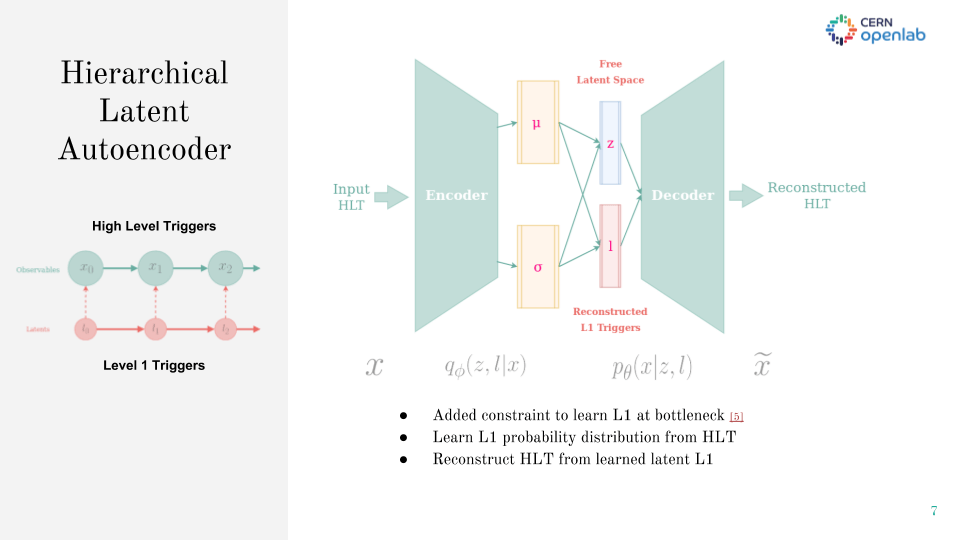
Well eventually, we successfully exploited the underlying hierarchical design of the CMS trigger system to enable better knowledge representation in our custom architecture we call Hierarchical Latent Autoencoder. None of this would be possible without the support and guidance of my supervisors: Adrian Alan Pol and Gianluca Cerminara. I am indebted to their reviews and advice because they taught me how to conduct and present my research work with accuracy, rigour and the true scientific spirit.
For this summer was not just about research, lectures and learning, but profound growth. It was not just events, visits and trips, but cherished memories. And this was not just an international internship, but a one-of-a-kind experience only CERN can provide.
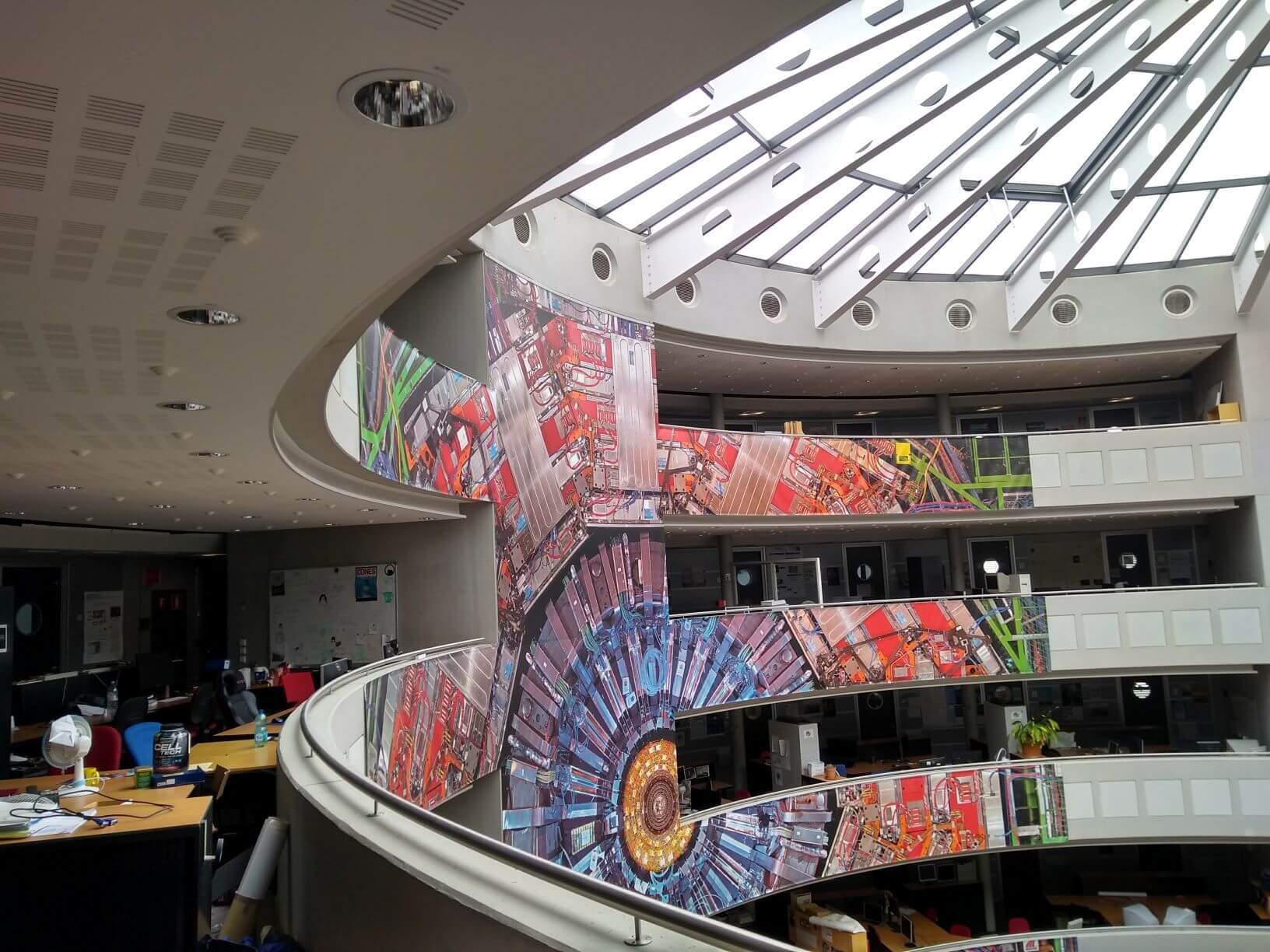
To dive in depth, here is my report and my 5-minutes Lightning Talk presentation. More on my website: amanhussain.com. Find me on twitter.